AI Agents and the Reality Check on Adoption: A Product Marketer’s Take
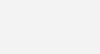
AI agents have become the tech world’s favorite buzzword. From autonomous support bots to generative AI copilots, the hype is loud and everywhere. There’s talk of revolutionary gains in efficiency, smarter operations, and totally reimagined workflows. Startups, enterprises, and everyone in between are jumping into the conversation, eager to claim a stake in what’s being called the future of work.
But let’s be real. While the excitement is warranted, the path to meaningful adoption is a whole different story.
As someone knee-deep in research and product marketing for AI solutions, I’ve seen the good, the great, and the very messy sides of this space. There’s no question that AI agents are game-changing, but unlocking their value at scale takes a lot more than just plugging them in. In this piece, I want to break down the promise, the friction, and why the biggest differentiator isn’t just technology—it’s data.
The Promise of AI Agents
AI agents, powered by machine learning, NLP, and automation, are already showing their potential across a range of use cases:
- Customer Support: Chatbots and virtual assistants are cutting response times, reducing support costs, and improving user experience.
- Business Operations: Repetitive tasks are being automated, freeing up human teams for more strategic work.
- Personalization: AI engines surface hyper-relevant recommendations and experiences based on real-time behavior.
- Data Insights: AI tools can comb through massive datasets in seconds to generate insights that would take humans weeks.
These aren’t just hypotheticals. Real companies are seeing real gains. But for every success story, there’s a reality check waiting on the other side.
The Enthusiasm vs. Reality Gap
Despite the noise, AI agent adoption is still patchy. Here’s what’s holding it back:
- Hesitation to Commit
AI doesn’t come cheap. Between engineering time, infrastructure, and unknown ROI, many companies are intrigued but unwilling to go all in, especially when the business case feels unproven. - It’s Not Plug and Play
There’s a misconception that AI is just another SaaS tool. It’s not. Behind the curtain, it demands solid data infrastructure, integrations, and constant tuning. If a company’s systems aren’t ready, AI becomes a burden, not a benefit. - Data is a Mess
You can’t run great AI on bad data. And yet, many organizations still deal with fragmented, siloed, or poor-quality datasets. Without a clean, connected foundation, even the most advanced AI agent can’t deliver. - Change is Hard
AI adoption often means changing workflows, roles, and even team mindsets. That’s a heavy lift, especially in companies where trust in AI is still shaky. - Regulation is Looming
Privacy concerns and ethical scrutiny are growing fast. No one wants to be caught on the wrong side of a compliance issue, and that’s making some companies cautious.
Innovation is Outpacing Adoption
AI innovation isn’t slowing down. OpenAI, Google, and others are making weekly headlines with new capabilities. But here’s the irony. The tech is sprinting while the market is still jogging.
Many businesses are still asking the basic questions: Do we need this? Is now the right time? Are we ready? This leaves product marketers in a tough spot. You’re promoting something revolutionary, but your audience might not be ready to hear it yet.
Why Data is the Real Differentiator
If there’s one thing I’ve learned through this work, it’s that technology alone doesn’t move the needle. Data does. Without high-quality, accessible, and constantly evolving datasets, even the smartest AI will struggle to deliver value.
- Garbage In, Garbage Out: Poor data leads to poor outcomes. It’s that simple.
- Silos Kill Performance: If your AI can’t access the right data across teams or systems, it’s going to underperform.
- Data Work is Ongoing: AI isn’t a one-and-done project. It thrives on updated, diverse, and relevant inputs.
Before betting on AI, organizations need to do the unglamorous but critical work of building solid data pipelines and breaking down silos.
What Product Marketers Can (and Should) Do
In this space, product marketing isn’t just about shiny feature lists. It’s about creating clarity and helping buyers get to a place where they can actually benefit from the innovation. That means:
- Leading with Insight: Ground your messaging in research and real-world outcomes, not buzzwords.
- Educating Thoughtfully: Teach your audience what AI can and can’t do. Set realistic expectations.
- Framing for Impact: Position AI as a long-term investment, not just the latest thing.
- Being Human: Acknowledge the complexity. Address concerns head-on. Empathize with where your buyers are on the adoption curve.
Closing Thoughts: Progress Over Perfection
AI agents are changing the game, but not overnight. Adoption will take time, and that’s okay. What matters now is how we guide that journey.
As builders, marketers, and evangelists, our job isn’t just to sell the dream. It’s to help companies build the runway to get there. If we can do that with empathy, honesty, and clarity, then AI won’t just be a shiny idea. It’ll be a force for real progress.
So the question isn’t whether AI is ready. It’s whether we are.